How can computer algorithms revolutionize the study of proteins and protein folding today? Click here to learn about the AlphaFold 2 algorithm!
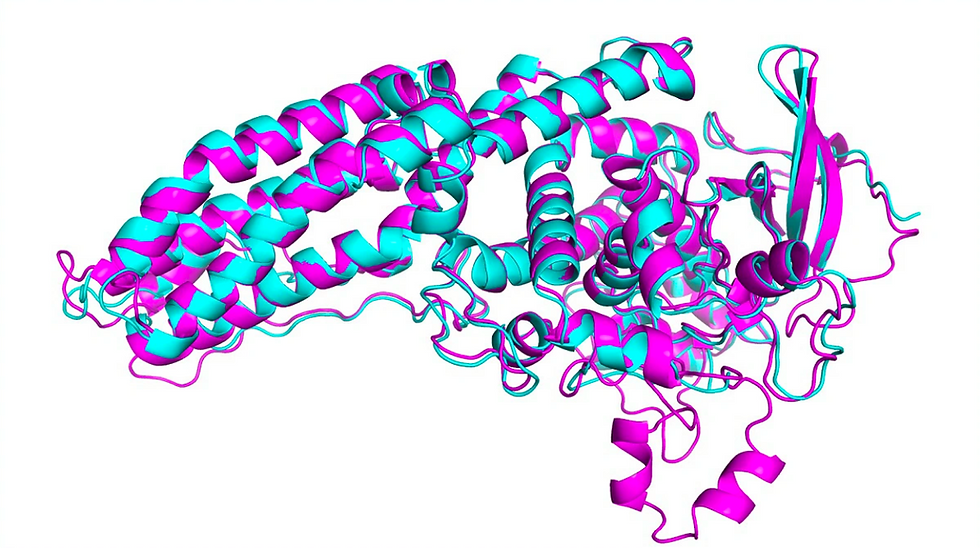
Figure 1: A protein
Introduction
When we think about proteins, we recall the ones inside our food and muscles. While true, proteins are, more specifically, microscopic molecules inside of the cells that carry out the diverse and vital biological functions of the body. A protein’s function depends on its shape, and when protein formation goes wrong, the resulting misshapen proteins cause problems that range from bad, when proteins neglect their important function, to significantly worse, when they cause extensive cell and tissue damage. Protein misfolding is a common cellular event that can occur throughout the lifetime of a cell, caused by different events including genetic mutations, translational errors, abnormal protein modifications, thermal or oxidative stress, and incomplete complex formations. If misfolded proteins linger in the cell, proteasomes – protein complexes that degrade unneeded or damaged proteins – recycle proteins and spit them out small fragments of amino acids, allowing the cell to reuse amino acids to make proteins.
Some misfolded proteins, however, are able to evade proteasomes which contribute to the pathology of many neurodegenerative diseases. This phenomenon is at the forefront of research for biochemists and biophysicists seeking to understand the great assortment of protein shapes and what misfolded shapes proteins can take on. DeepMind’s protein-folding AI, AlphaFold, has predicted these misfolded shapes to within the width of an atom in a scientific breakthrough of the century, but to understand this breakthrough, we need to delve more into the work of biophysicists.
Biophysics and Protein-Folding
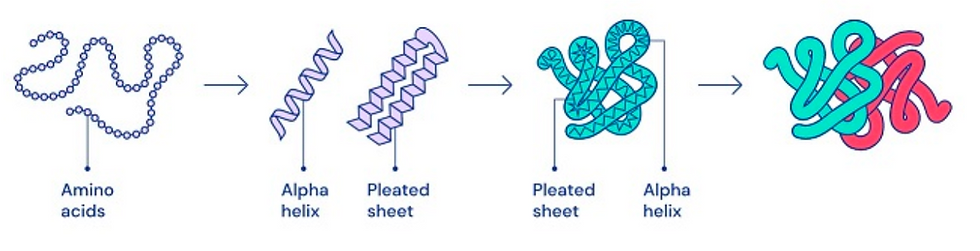
Figure 2: The levels of protein folding
In Biophysics, – a field that applies the quantitative theories and methods of physics to study biological systems – scientists hope to use quantitative tools to predict vast assortments of misfolded proteins. This field is also known as the "bridging science" between biology and physics, as physical scientists use mathematics and computer simulations to understand how biological systems work. In addition to protein and structural biology, these biological systems include cells, organisms, and entire ecosystems. Thus, biophysicists, work to develop methods to overcome disease, eradicate global hunger, produce renewable energy sources, design cutting-edge technologies, and solve countless scientific mysteries at the forefront of solving age-old human problems that can eradicate us as a species.
Interestingly, many biophysicists today are running computer simulations to predict various shapes of proteins. Predicting the assemblies of these misfolded proteins, however, is no easy feat. With the help of machine learning, researchers can more easily design new drugs and understand diseases thanks to the computing power of machine learning algorithms.
Biophysicists require high computing power, as there are over 100,000 unique types of protein with a wealth of diversity and specificities in each of their functions. Protein structure prediction is notoriously difficult because a polypeptide is very flexible, and has the ability to rotate in multiple ways at each amino acid, causing it to fold into numerous different kinds of shapes.
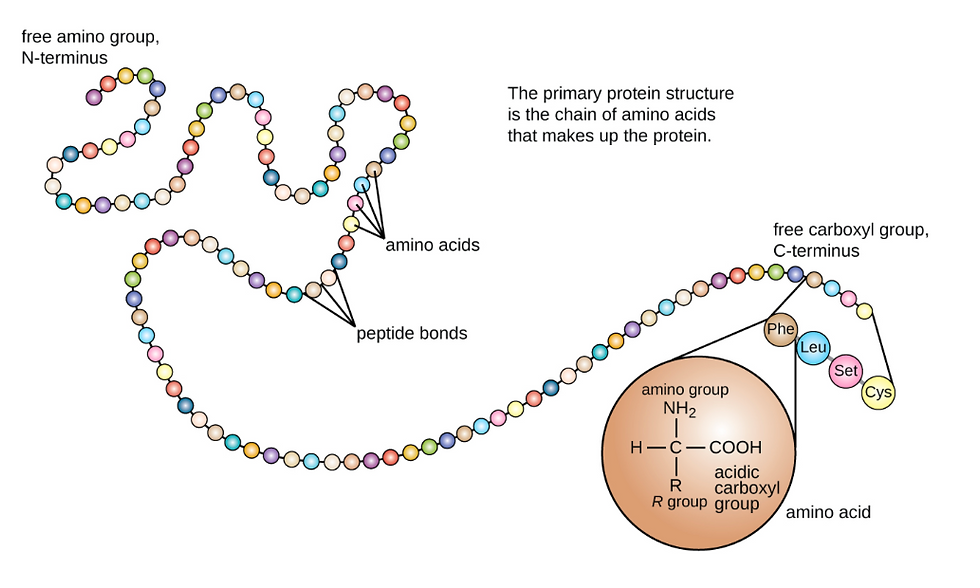
Figure 3: A diagram of the primary structure of a protein
Proteins are also unpredictable when just going off of the amino acid sequence (Which is the arrangement of the basic building blocks in proteins and peptides). All the information needed for a protein to fold into its three-dimensional conformation is contained in the amino acid sequence. While some proteins usually follow the correct path according to their amino acid sequence, others take a detour that can make them fold into very different and useless structures.
Furthermore, it can be even more difficult to predict protein structure from an amino acid sequence because small perturbations in the sequence of a protein can drastically change the protein’s shape and even render it useless. Additionally, different amino acids can have similar chemical properties, and so some mutations will hardly change the shape of the protein. As a result, two very different amino acid sequences can fold into proteins with similar structures and comparable functions.
Despite the issues of predicting proteins, DeepMind’s AlphaFold AI startled many scientists in the field with its gigantic leap in approach to these polypeptide structures.
DeepMind
Since 1994, the Critical Assessment of protein Structure Prediction (CASP) was founded with the goal of helping to advance the methods of identifying protein structure from the amino acid sequence. The event challenges teams of scientists to predict the structures of proteins that have been solved using experimental methods. DeepMind’s performance in 2018 at CASP13 startled many scientists in the field with its approach. In particular, DeepMind’s AlphaFold AI takes in structural and genetic data to predict the distance between pairs of amino acids inside of a protein. AlphaFold then uses this information to come up with a general model of what the protein should look like according to the structural and genetic data.
The team eventually hit a wall with this approach, so they incorporated additional information about the physical and geometric constraints that determine how a protein folds. They also looked to change their approach from determining the relationship between amino acids to determining the final structure of a target protein sequence.
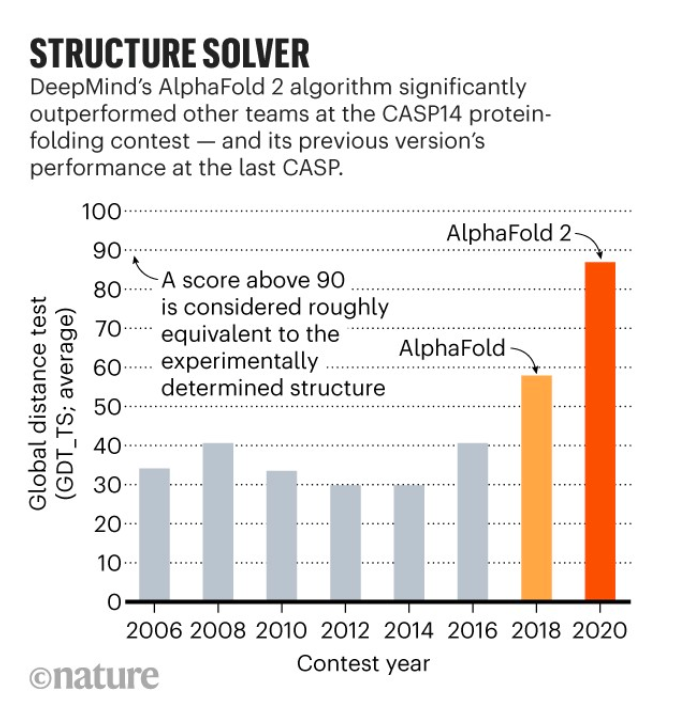
Figure 4: A diagram detailing the massive efficiency and performance of the computer algorithm AlphaFold 2 at the CASP14 protein folding contest.
In CASP, results are scored using what’s known as a global distance test (GDT), which measures - from the values 0 - 100 - how close a predicted structure is to the actual shape of a protein identified in lab experiments. Eventually, over the course of several months of the event, the latest version of AlphaFold scored well for all proteins in the challenge. But it got a GDT score above 90 for around two-thirds of them. Its GDT for the hardest proteins was 25 points higher than the next best team, says John Jumper, who heads up the AlphaFold team at DeepMind. In 2018 the lead was around six points. AlphaFold, as a result, far outstripped all other computational methods and for the first time matched the accuracy of techniques used in the lab, such as cryo-electron microscopy, nuclear magnetic resonance, and x-ray crystallography. These "lab-work" techniques, however, are expensive and slow: it can take thousands of dollars and years of trial and error for each protein. AlphaFold, however, can find a protein’s shape in a couple days.
What’s Next?
AlphaFold builds on the work of hundreds of researchers around the world. DeepMind also draws on a wide range of expertise, putting together a team of biologists, physicists, and computer scientists. Details of how it works have been released in a peer-reviewed article in a special issue of the journal Proteins in 2021.
Today, many drugs are designed by simulating their 3D molecular structure and looking for ways to slot these molecules into target proteins. Of course, this event can only be done if the structure of those proteins is known. This is the case for only a quarter of the roughly 20,000 human proteins, leaving 15,000 untapped drug targets for AlphaFold and other biophysicists, biochemists, and computational biologists to explore in a new area of research.
DeepMind says it also plans to study leishmaniasis, sleeping sickness, and malaria, all tropical diseases caused by parasites, as they are linked to multiple unknown protein structures. Ultimately, AlphaFold had revolutionized computational science compared to traditional methods such as Monte Carlo integration and other computational techniques. Who knows, potentially someday similar technology can be applied to solve other issues in physics and synthetic chemistry.
Citations:
Hashtags: #biology #NowScience #Sciencebehindit #current #NowScience #Blog #Interestingtopic #science #scienceresearch #theories #personalopinion #proteins #aminoacids #AlphaFold #DeepMind #biophysics #algorithms
コメント